Concerns and Risk of Artificial intelligence (AI) in Health care

Artificial intelligence (AI) rapidly dominates the health service system and serves major roles, from automating drudgery and routine tasks in medical practice to managing patients and medical resources. It removes the manual health system into automatic, in which humans conduct the routine works/tasks in medical practice to the management of patients and medical resources. As developers create AI systems to take on these tasks, several risks and challenges emerge, including the risk of injuries to patients from AI system errors, the risk to patient privacy of data acquisition and AI inference, and many more…!
The health service sector needs innovative solutions to find out how to be more effective and efficient without excessive expenditure. This is where technology comes in for the solutions. Rapid developments in technology, especially in the fields of AI and robotics, can assist the complement of the healthcare industry. Potential solutions are complex but involve investment in infrastructure for high-quality, representative data; collaborative oversight by both government agencies and other health-care actors; and changes to medical education that will prepare providers for shifting roles in an evolving system.
Questions arise whether AI can exercise doctors’ rights and obligations, protect privacy issues, and the applicable law is not fully prepared with this progress. The use of AI for the healthcare system in the world indicates that current regulations support it. It is proven that the rules in the development of technology and health technology products can be developed and applied for medical care.
Bias and inequality is one of the major concerns.!
There are risks involving bias and inequality in health-care AI. AI systems learn from the data on which they are trained, and they can incorporate biases from those data. For instance, if the data available for AI are principally gathered in academic medical centers, the resulting AI systems will know less about a special medical condition and therefore will treat less effectively the patients from populations that do not typically frequent visits to academic medical centers. Similarly, if speech-recognition AI systems are used to transcribe encounter notes, such AI may perform worse when the provider is of a race or gender underrepresented in training data
How Safe AI Technologies in healthcare..?
A primary limitation in the development of effective AI systems is the caliber of data. Many AI models rely on training data that reflect the “ground truth,” a best-case scenario in which researchers know the outcome in question, based on direct observation. Retroactively establishing the “ground truth” requires careful clinical review and annotation, which is a time- and resource-intensive process. However, the “ground truth” is not always easily determined. Clinicians may interpret cases differently or assign different labels for broadly defined conditions, leading to poor reproducibility. Errors in AI systems can cause injury. For example, through Incorrect recommendations, recommendations based on false-negative or false-positive results .Model resilience, or how an AI technology performs over time, is a related risk.
An AI application can provide the wrong guidance if it contains code errors due to human programming mistakes. It is possible that a developer designs an AI technology unethically, to produce an outcome that would generate profits for the provider conceal certain practices. Malicious design has affected other sectors, such as the automobile sector, in which algorithms used to measure emissions were programmed to conceal the true emissions profile of a major car manufacturer. Use of computers carries an inherent risk of flaws in safety due to insufficient attention to
1.Minimizing risk in the design of machines
2.Bugs or flaws in program code
3.Quality of data sets used to train algorithms
Injuries and deaths due to such flaws are underreported, and there are no official figures and few large-scale studies. As health care systems become increasingly dependent on AI, these technologies may become targets for malicious attacks.
For example, a system could be hacked to shut it down, Manipulate the data, ”Kidnap” data for ransom.AI developers might be targeted in “spear-fishing” attacks. An algorithm could be hacked to generate revenue for certain recipients, and large sums are at stake. Health data is some of the most sensitive data about individuals. Security breaches could harm, Privacy, Dignity, Human rights.

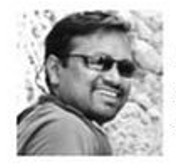
Author –Ragesh R
IT professional specialized in healthcare technologies with over two decades of experience. He also has a fondness for photography, traveling, designing, painting, and sharing knowledge.